DTU
ESR 1
|
|
PhD title:
Digital Manufacturing for Extrusion 4.0
|
Arhimny holds a Master Degree in Smart Systems Integration which is an Erasmus Mundus Joint Master Degree in three participating universities: Heriot-Watt University (Edinburgh, Scotland, UK), University of South-eastern Norway (Vestfold, Norway), and Budapest University of Technology and Economics (Budapest, Hungary). Before pursuing her master's degree, she worked in Indonesia as Instrument and Control Engineer in Project Development where she was involved in several projects related to design engineering, construction, commissioning, start-up, and operation.
Polymer extrusion is a well-established technology due to its high productivity and the ability to manufacture very complex cross-section products. However, for highly customized products and small batches, the die design and manufacturing are lengthy processes that limit new products development and their production. Therefore, the opportunity is foreseen to integrate Additive Manufacturing, Process Simulation, and Digital Dataflow into the conventional manufacturing platform to overcome these limitations and to innovate the extrusion process and value chain.
The main objectives of PhD projects are:
- Integrate product/process/die design engineering by using multi-physics simulations of the extrusion process (extrusion digital twin).
- Develop additive manufacturing technologies for the rapid direct manufacturing of extrusion dies.
- Establish an extrusion pilot production with Additive Manufactured dies and in‐line product/process monitoring.
|
DTU
ESR 2
|
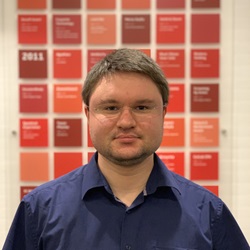
|
PhD title:
Integrated digital manufacturing technologies for the production of polymer micro components
|
Industry4.0 precision injection moulding production of polymer micro components is becoming more and more important.
The potential benefits arise from digital integration of process chain. This means intelligent connection of manufacturing step and subsequent quality control step in order to stabilize the precision injection moulding process, reduce discard of polymer micro components, and improve part quality.
Furthermore, Additive Manufacturing (3D Printing) has the potential to disrupt conventional tooling business engaged in supply of precision injection moulds for polymer micro component manufacturing. The application of additively manufactured tools has the potential to reduce lead time and costs of prototyping moulds and thus decrease time and costs needed for development of new polymer micro components. In consequence, integration of additively manufactured precision injection moulds may enable producers of injection moulded polymer micro components to reduce the time-to-market and to cut development costs of new products.
|
DTU
ESR 3
|
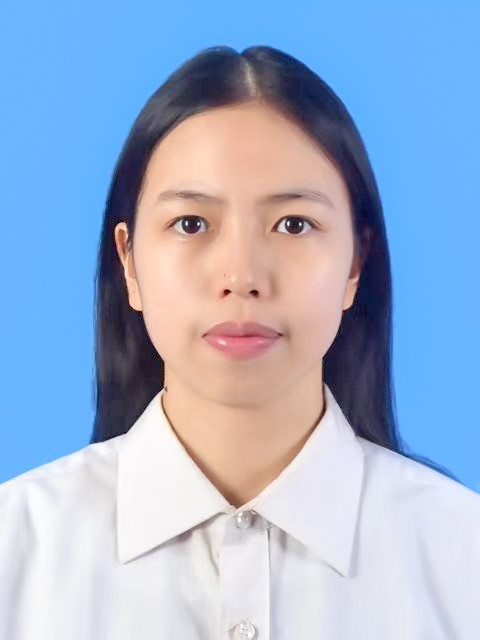 |
PhD title: Improve overall equipment efficiency in crystal growth production |
Tingting held a Master’s degree in Mechanical Engineering from Tsinghua University.
As a crucial component in many electronics, semiconductor silicon wafer becomes building block for all modern technology. In the production of wafer, crystal growth is an important step to obtain single-crystal silicon. Float zone process, a method for growing single-crystal silicon, is by far the most pure method and can result perfect silicon. However, float zone process is suffering from low Overall Equipment Efficiency. This PhD project’s goal is to develop, apply and validate specific digital manufacturing technologies to solve the problem.
The main objectives of the PhD project are focused on the following aspects:
• Develop an automatic model with general solution of process parameters for different process environment including different process configurations and different float zone machines.
• Develop an analytical model for predicting the performance of float zone machines , so as to reduce downtime and increase availability of machines.
•Integrate autonomous robots into the system to reduce number of failures caused by human errors.
|
DTU
ESR 4
|
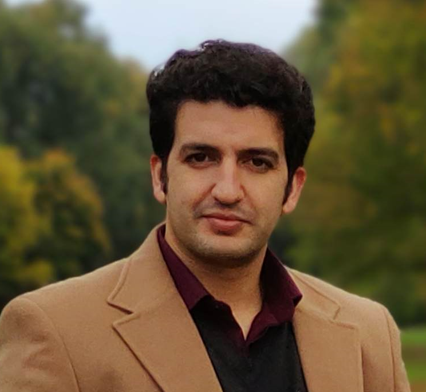 |
PhD title: Digital Moulding 4.0 - Advanced Process Analytics for Zero-Defect Injection Moulding Technology |
Alireza holds a master’s degree in mechanical/Manufacturing Engineering. Before joining Technical University of Denmark, he started his research on plastic injection molding process and application of data analysis and machine learning in defect prediction.
With increasing use of plastic products all around the world, it becomes more and more important to have high quality products and avoid defects as much as possible. There are a variety of injection defects and numerous factors that can be influential on each defect. To avoid defects, it is important to know which parameters can affect which defects, how, and how much?
The main objectives of the Ph.D. program can be summarized as follows:
- Creating digital twins for injection molding process
- Data analysis using both machine learning and statistical methods in the context of Industry 4.0.
- Training prediction models to speed up getting the results of an undone injection process.
|
KIT
ESR 5
|

|
PhD title:
Digital Production Control through Artificial Intelligence infused Industry 4.0 for real-time like planning and scheduling
|
Production Planning and Control (PPC) is the art of managing an ever increasing complexity in the manufacturing of products. A novel approach to enable concurrent production and merge Job Shop and Line production is a matrix design inspired type of manufacturing: Matrix-production. Managing such complex production systems requires new and intelligent PPC, in contrast to currently applied heuristically developed approaches. My research focusses on the development and application of artificial intelligence for this domain. Beyond the algorithmic work itself the integration into the productions IT infrastructure and its development is a relevant area of my research. Hence, the main goal is to develop and validate intelligent PPC as one way to make use of the often suboptimal PPCs potential. Prior to joining wbk&KIT, I have been studying Industrial Engineering and Management and working with Industrial partners (Automotive, Semiconductor, …) and universities abroad (US, China, France,…) alike.
|
UNI ZAR
ESR 6
|
|
PhD title:
Development of innovative material flow methods for lean assembly in Industry 4.0 production |
Edoardo obtained a Master's and a Bachelor's degree in Mechanical Engineering at the University of Pisa (Italy). After the graduation he worked for two companies in Italy (Letomec S.R.L and Valiani S.R.L.) as a design engineer, on the development of a new gripper and a cutting head for plotters. During these works and his Master thesis he dealt with machine component design, FEM and Dynamic analysis, Automatic control and simulations. Now he is actually enrolled in a PhD program in the field of Development of innovative material flow methods for lean assembly in Industry 4.0, at the University of Zaragoza.
The objectives of this PhD project can be summarized as follows:
• Development and simulation of novel modular grasping, feeding, sorting and conveyor systems for in time manipulation of material flows.
• Development of control and monitoring environment employing visual object detection.
• In-line integration in assembly process and system optimization.
|
UNI ZAR
ESR 7
|
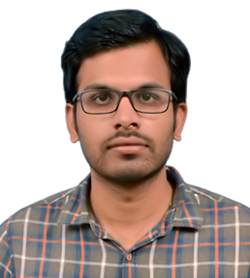
|
PhD title:
Deep learning algorithms in Industry 4.0 Quality Control for real time machine vision
|
I have been working at TATA research center for more than six years and my research projects were related to Computer vision, Deep neural networks, Machine learning and artificial intelligence in Industry 4.0 application. My nature of work involves acquiring, processing, analyzing and understanding digital images, and extraction of high-dimensional data from the real world in order to produce numerical or symbolic information, e.g., in the forms of decisions.
The primary focus of my PhD project will be to investigate and develop a method for automatic defect identification in ferromagnetic parts based on Computer Vision and Deep learning techniques. In the case of ferromagnetic parts production, magnetic particle inspection allows detecting some surface and near-surface defects which are not otherwise visible to the naked eye. The identification of these defects is done by qualified operators by visual inspection of the parts once the magnetic particles are applied. The proposed approach is to combine basic image analysis with state-of-the-art deep learning techniques in order to improve the defect detection accuracy.
|
UNI ZAR
ESR 8
|
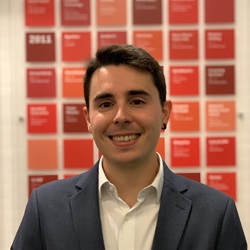
|
PhD title:
Industry 4.0 technologies for high product customisation and high assembly productivity in white goods low volume production. |
Changes in demand patterns suggest that mass customization will be a major challenge for white good manufacturers since they need to remain competitive while producing a large total volume of fundamentally similar yet different products (high mix low volume).
Existing high volume, highly automated production systems lack the necessary flexibility to address this new demand trend.
Industry 4.0 novel technologies such as collaborative robots, augmented reality and in-line Quality Control are promising research areas which could be used along with Lean Manufacturing methodologies in order to improve assembly operations.
This line of research focuses on developing methodologies to assess and implement Industry 4.0 technologies to improve high mix – low volume assembly processes in terms of flexibility, quality and productivity.
Adrian obtained his BSc and MSc degrees on Industrial Engineering at University of Zaragoza, Spain. He worked for IMI Precision Engineering in the UK for three years before being awarded a PhD scholarship to join the Department of Design and Manufacturing at the University of Zaragoza.
|
UNI ZAR
ESR 9
|
 |
PhD title:
Industry 4.0 Quality control by non-contact 3D Metrology using Digital Manufacturing Technologies |
My current project, which is conducted within the Doctorate program of Design and Manufacturing Engineering at University of Zaragoza, focuses on developing of an application for quality control by non-contact 3D metrology using cutting-edge Digital Manufacturing Technologies like Augmented Reality (AR), Big Data, etc. to achieve the innovative technological solution for high quality, high throughput and high precision production.
In the previous master program, I supported the final review of the international project on “Learnstruments in Value Creation Networks” relating to the framework of the Collaborative Research Center 1026 (CRC1026) “Sustainable Manufacturing” between Technical University of Berlin (Germany) and Vietnamese-German University (Vietnam). I had been selected for a scholarship to conduct my master thesis at TU Berlin in 2016 and successfully achieved the master degree granted by TU Berlin.
I prefer participating in multi-disciplinary projects, that I could have opportunities to work with emerging technologies as well as enhance my skills in combining various methodologies of different fields to develop innovative solutions for those challenges.
|
POLIMI
ESR 10
|
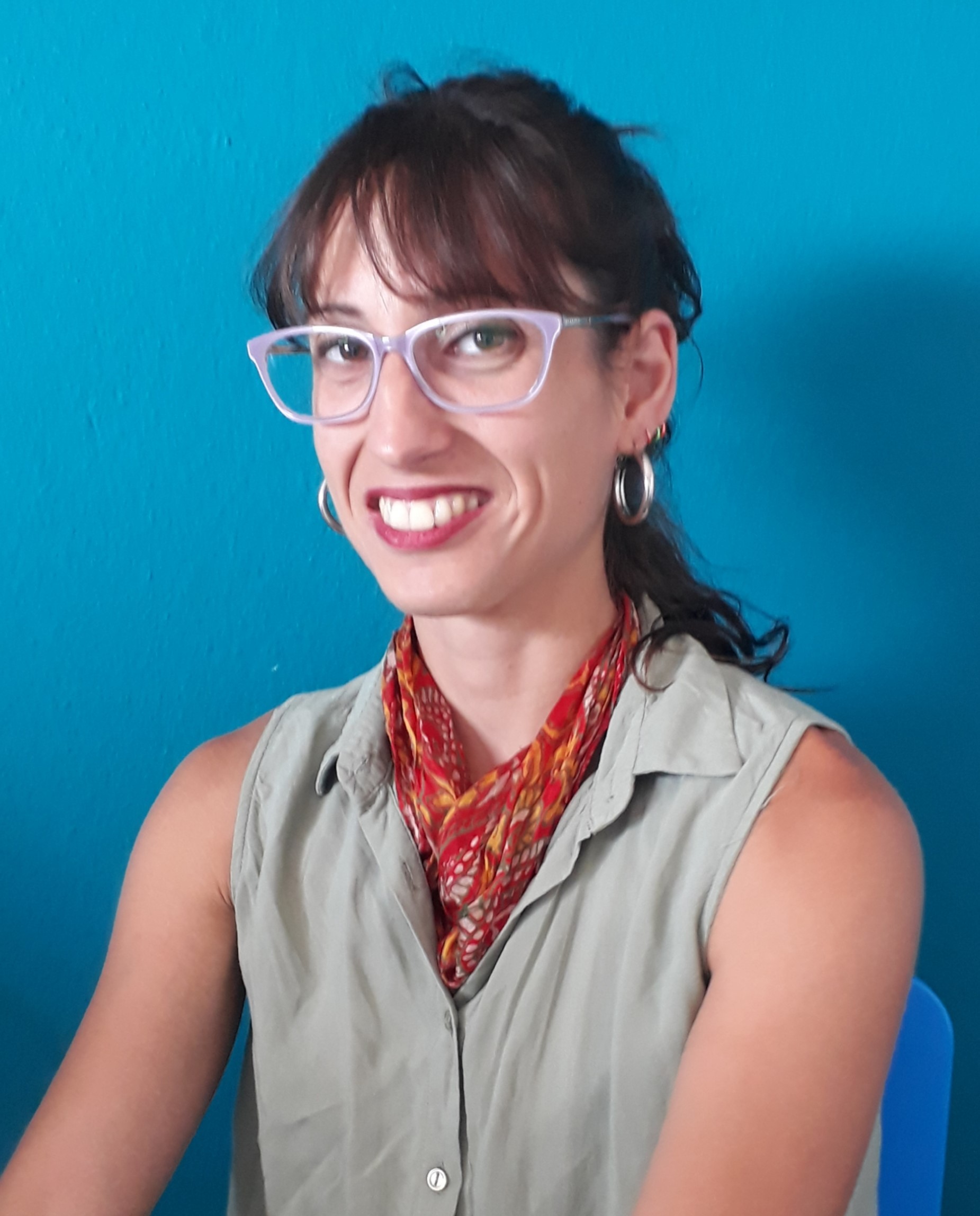 |
PhD title:
Cyber‐physical system for feed‐forward and quality‐oriented assembly of complex aerospace parts in Industry 4.0 production
|
Catherine studied Mechanical Engineering and Aeronautics at the University of Patras (Greece) and graduated with a Master's degree with focus in the field of Design and Manufacturing. During her studies she was working as a part-time research assistant at EU funded projects and after graduation she worked in the industry around Europe and has experienced the challenges of big international companies with their own production lines. Her PhD in the Politecnico di Milano is linked with the development of CPS in Industry 4.0 production. Cyber-Physical Systems (CPS) are a new generation of systems that integrate computational and physical capabilities to a system that is able to interact with humans in many new modalities
Her objectives regarding the project are the following:
• To develop a new feed-forward quality-oriented assembly system enabling to adapt the assembly strategy depending on the specific quality characteristics of the input components, simultaneously achieving a reduction of assembly times and an improvement of assembled product quality.
• To implement this solution and the related technologies in a new Cyber-Physical System. This system will be composed of an in-line data gathering system, data-analytics algorithms for critical product feature extraction and for in-line assembly strategy optimization, and a decision support system to visualize to the operators the parts to be assembled and the specific assembly strategy.
• To build and validate a demonstrator showing the benefits of this system at the facilities of the industrial partner. |
POLIMI
ESR 11
|
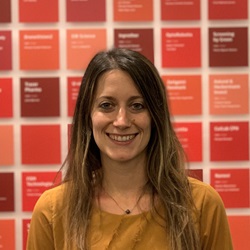
|
PhD title:
Automatic process planning and design of manufacturing cells in Industry 4.0 production
|
Beatriz holds a Master's Degree in Industrial Engineering and a Master in Biomedical Engineering from University of Zaragoza. Before joining Politecnico di Milano she worked in different automation companies in the United Kingdom, where she could test the issues that companies suffer not only when they decide to embrace the new digital transformation but also when they decide to remain as they always were, due to several fears such as investment, training, mindset, etc..
The main objectives of her thesis are focused on three aspects:
- Definition of a method to standardise to the available information on machines, tools, fixtures and operators’ experience.
- Development of a comprehensive process planning approach capable of managing and link information on the involved resources, manufacturing operations and parts also considering the operators’ knowledge.
- Introduction of cyber physical simulation of processes and relevant signal monitoring capabilities to improve the process chain knowledge.
|
POLIMI
ESR 12
|

|
PhD title:
Risk-based machine scheduling in the manufacturing systems in Industry 4.0 production |
Lei holds a Master Degree in Industrial Engineering from Tsinghua University, China. Before joining Politecnico di Milano, he worked in different software companies in China, where he could apply operations research, machine learning and software engineering skills to solve the financial and transportation problems.
His PhD project has the following objectives:
- Design and management of manufacturing systems which are combined with the most advanced methodologies for performance evaluation, planning and scheduling under uncertainty, simulation, and optimization.
- Construction of models of the uncertainty affecting the manufacturing process.
- Development of risk-based scheduling approaches to manage the intrinsic uncertainty in the manufacturing and remanufacturing activities, Specifically, the approaches are focused on the re-manufacturing of blades during the maintenance and/or refurbishing of the turbines.
- Building and validation of a demonstrator showing the benefits of this system in a real manufacturing environment.
|
UNIPI
ESR 13
|
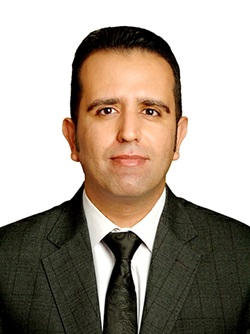
|
PhD title:
Vertical Integration in end‐to‐end product‐process value chain 4.0
|
Mohammad Amin held a Master's degree in Electronic Engineering in 2010. before joining the University di Pisa, he worked for SRTO (Shiraz Railway Transportation Organization), where he focused on the design and implementation of embedded based Industrial Automation systems for eight years. The main objectives of his thesis have focused on data gathering and data integration in Smart Industries 4.0.
Data collection plays a crucial role in supplying Automation systems. To implement a dynamic Automation Network, this information must contain practical, accurate, and delivered data to the recipient at the correct time.
To do this, the downstream bulk of the data collected must be fully refined and sent to the upstream layers after preparation and encryption of valuable data. In order to reduce the amount of information sent and received on Automation networks, it is vital to downsample the information precisely at the place of generation. Both upstreaming and down streaming will be thoroughly investigated and also their connection outside the company within the supply chain.
|
UNIPI
ESR 14
|
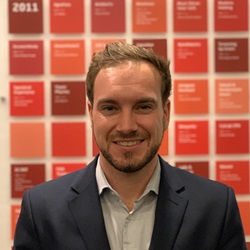
|
PhD title:
Development of innovative grasping methods for cobots sensitivity improvement in Industry 4.0 production |
Grasping devices and methods play a central role in automated production. They perform tasks such as assembling, handling, separating, positioning and packaging of parts, components and final products in almost every industry. Recent needs are pushing automation towards multifunctional grippers capable to achieve a variety of operations. Within this PhD project different methods and principles will be employed to develop grippers with increased flexibility and shape adaptability, including the application of compliant mechanisms. The research approach further focuses on practical applicability and acceptability investigated through the methods of pretotyping.
As gripper designs not only depend on object properties but also rely on previous and consecutive process phases, the research tends to industrial integrated robotic solutions with respect to IoT and Digital Manufacturing. Up-to-date real case problems will be continuously investigated in strong collaboration with industry partners. The cooperative work of operators and collaborating robots (cobots) will be especially emphasised.
|
UNIPI
ESR 15
|
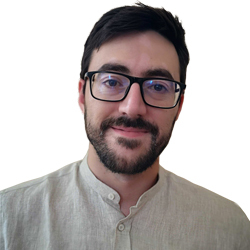 |
PhD title:
Embedded IoT solutions for Industry 4.0 manufacturing process digitalization |
Marko obtained his BSc and MSc degrees on Electrical Engineering at the University of Belgrade, Serbia. He worked for Mikroelektronika in Belgrade, Serbia, for five years before being awarded a PhD scholarship to join the Department of Industrial and Civil Engineering at the University of Pisa.
Industry 4.0 is the industry of the future. In order to make it the industry of today it is important to provide the industrial landscape with the means to adopt the Industry 4.0 paradigms efficiently and thoroughly. This PhD project’s goal is to provide a complete solution for this purpose.
The main objectives of the PhD project are:
• Development of microservices (“connectors” at the software level) with respect to PLCs on one side and MRP or ERP on the other side.
• Designing a system to balance the computational effort between node‐edge in different industrial cases and with/without broadband connectivity.
• Development of a generic structure of services (also by using or integrating services provided by other suppliers like Microsoft, IBM, etc..) to perform big data analysis, synthesis, and representation for production, maintenance, etc. |